You signed in with another tab or window. Reload to refresh your session.You signed out in another tab or window. Reload to refresh your session.You switched accounts on another tab or window. Reload to refresh your session.Dismiss alert
mitsuhiko-nozawa
changed the title
[WIP] Asynchronous Distributed Learning with Sparse Communications and Identification
Asynchronous Distributed Learning with Sparse Communications and Identification
May 28, 2021
一言でいうと
ローカルモデル(スレーブ)の更新をランダムにスパース化することによって、マスタモデルとのコミュニケーションコストを抑えた非同期学習手法。強凸な関数においては固定ステップ数で線形に収束することを証明。
論文リンク
https://hal.archives-ouvertes.fr/hal-01950120/document
著者/所属機関
Dmitry Grishchenko*, ranck Iutzeler*, J´erˆome Malick†, Massih-Reza Amini*
*Universit´e Grenoble Alpes
†CNRS, Laboratoire Jean Kuntzmann
投稿日付(yyyy/MM/dd)
December 10, 2018
概要
※identification(同定)とはおそらく、重みが非ゼロ要素となるインデックスを見つけ出すこと
データが中央集権的でなく、M個のマシンに分けて格納されている状況を考える(非共有メモリ)。
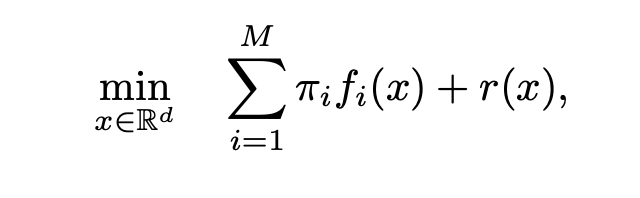
すると、目的関数が以下のように表せる。
iはマシン、πiはマシンiが持つデータの割合、fi(x)はモデルxでのマシンiが持つ観測データにおける経験リスク
非同期分散学習ではイテレーティブな最適化では優れた通信がボトルネックになるが、本研究では高レイテンシ、断続的な学習でも動作するようにしている
upward communications (slave-to-master) と downward communications (master-toslave)の両方をスパース化する。
それらの実現のために、
の3つのスパース化を用いる。
新規性・差分
非同期な学習手法の中でも、スレーブからマスタ、マスタからスレーブの両方でスパースな通信を行う手法は本手法が初めて。
提案手法が、遅延についての軽量な仮定の上でほぼ最適な部分構造(サポート)の同定を行うことを証明。
手法
sparsification
1.M個のマシンから1個選択
2.重みの中からランダムに更新対象を選択
3.選択された重みの持つ蓄積された勾配をマスターマシンに通信して送る
4.マスタの重み更新、集約重み計算、このイテレーションでの重み計算
5.スレーブマシンに重み渡す
※勾配計算の際に遅延した重みを使って計算している
Identification
l1正則化付き問題において、マスクSを用いて計算すべき重みを抽出する際、サポートは別で扱うようにする。
結果
ラッソ回帰とエラスティックネット付きのロジスティック回帰で実験
densityが減っている = サポートの同定ができていることを示す。
suboptimalityは何を意味しているのかわからなかったが、同じsuboptimalityで少ないdata exchangeなら良いということか
グラフから、fig1, fig2では提案手法がかなり勝るが、fig3では状況が一変している。mask size(サポート数)分ランダムに要素を選択するdaptiveな手法なら有効であった。
コメント
deep gradient compressionとは直接関係ないかもしれません…
The text was updated successfully, but these errors were encountered: